Introduction To Places_512_Fulldata_G.Pth 路径
Image inpainting has visible great improvements with the creation of the places_512_fulldata_g model, a present day tool designed to restore and enhance virtual snap shots. This model, housed within the Places_512_FullData_g.Pth file, has emerge as an vital useful resource for professionals and hobbyists alike. Its ability to seamlessly reconstruct missing or broken regions of pix ensures sensible and accurate consequences, making it a treasured asset throughout industries like pictures, digital art restoration, and innovative design. The version sticks out for its capacity to interpret the encircling context and generate coherent, wonderful content, raising the requirements of photograph inpainting.
This guide will take a deep dive into the functionality and application of the places_512_fulldata_g model for photograph inpainting. We will explore the structure and running principles of the model, detailing the way it approaches visible records and is familiar with contextual records to supply herbal-looking inpainted regions. Furthermore, the guide will offer vital suggestions on getting ready pics to gain most beneficial inpainting outcomes, ensuring that customers could make the maximum of this effective device.
Additionally, readers will study techniques to decorate the overall performance of the model for the duration of the inpainting technique. This includes realistic recommendation on configuration settings and techniques to streamline workflow and maximize output fine. By following this complete guide, users will advantage the expertise and skills wished to make use of places_512_fulldata_g efficaciously in their innovative projects, starting up new avenues for photo recovery and enhancement.
The places_512_fulldata_g model represents a significant development in digital image restoration and inpainting. Built on an advanced adaptation of Stable Diffusion, this model has been specifically optimized for the task of filling in missing or damaged areas of photos, achieving impressive levels of accuracy and detail. The core file for this model, places_512_fulldata_g.pth, is essential for anyone looking to harness its powerful inpainting capabilities.
Model Architecture and Technical Foundation
The places_512_fulldata_g model is built upon the Stable Diffusion 1.5 architecture but has undergone specialized modifications to enhance its performance for image inpainting. The training process comprises two distinct phases: an initial 595,000-step general training phase followed by 440,000 additional steps focused on inpainting, all using a resolution of 512×512 pixels. This layered training approach ensures the model can effectively interpret complete images and understand how to best reconstruct masked or missing areas.
Core Architecture and Features
At the heart of the places_512_fulldata_g model lies an adapted UNet architecture. Unlike standard models, this version includes five extra input channels. Four of these channels carry the encoded image data, while the fifth represents the mask that outlines the inpainting area. This enables the model to process the existing image while considering what needs to be reconstructed.
Key components of the model include:
- Input Layer: Accepts the original image along with its mask.
- Encoding Layers: Compress and process the image data.
- UNet Core: Central layer where inpainting computations are performed.
- Decoding Layers: Upsample and refine the output for clarity.
- Output Layer: Delivers the completed image.
Advantages Over General Image Generation Models
The places_512_fulldata_g model has distinct advantages when it comes to inpainting, setting it apart from more general image generation models:
- Contextual Insight: Trained specifically on both full and masked images, this model excels in understanding the context of the existing image, making it better at seamless integration.
- Smooth Edges: The model minimizes the visibility of boundaries where inpainting occurs, yielding a more natural and cohesive look.
- Enhanced Prompt Comprehension: The model responds more accurately to user instructions, creating contextually appropriate additions.
- Outpainting Functionality: Beyond just inpainting, it can extend images beyond their original size, providing flexibility in creative projects.
Preparing Images for Effective Inpainting
Proper image preparation is crucial for achieving the best results with the places_512_fulldata_g model. Here are essential steps and techniques to consider:
Image Preprocessing
- Resizing: Uniform resizing to 512×512 pixels is critical as it aligns with the model’s training specifications, allowing the algorithm to process the data efficiently.
- Normalization: Adjusting pixel intensity to a range of 0 to 1 improves model performance and consistency.
- Contrast Enhancement: Techniques such as histogram equalization can improve image quality, ensuring better inpainting outcomes.
Mask Creation Tips
Creating precise masks is an integral part of the inpainting process. The use of image editing tools allows for accurate masking, where the mask blur slider can be adjusted to control the sharpness of the mask edges. Higher values offer smoother transitions, ensuring a seamless blend with the original image. For intricate details like hands or facial features, enabling “inpainting at Full Resolution” allows for more detailed output.
Handling Different Resolutions
Though trained primarily at 512×512 pixels, the places_512_fulldata_g model can be adapted for higher resolutions, even up to 2k. This flexibility lets users maintain quality without significant detail loss, though processing time may increase with larger images. Balancing resolution and processing efficiency is crucial for achieving optimal performance.
Performance Optimization Strategies
To maximize the model’s performance, optimizing GPU usage is essential:
- Mixed-Precision Training: Using a combination of 32-bit and 16-bit floating-point types can reduce memory consumption and speed up processing while maintaining output quality.
- Data Transfer Efficiency: Faster data movement from the CPU to GPU can be supported by employing data caching and using CPU-pinned memory.
- NVIDIA DALI Library: This tool helps build optimized data pipelines, offloading specific preprocessing tasks to the GPU for smoother operation.
Balancing Speed and Quality
For high-quality results, careful consideration of printing settings, such as layer height, is recommended. A range of 60–100 microns often yields good detail without overly extended processing times. While detailed print settings can extend times, they can also enhance output clarity and minimize potential issues like warping.
Post-Processing Techniques
To further refine the results produced by the places_512_fulldata_g model, post-processing can be invaluable:
- Denoising: Techniques such as Gaussian blur or median filters can reduce noise and improve clarity.
- Avoid Certain Techniques: Methods like binarization and erosion may not effectively improve the overall quality and should be used with caution.
- Combining Methods: Layering multiple post-processing techniques can enhance results, though it’s important to test and confirm the benefits for specific use cases.
By following these strategies for preparation, image handling, and optimization, users can maximize the capabilities of the places_512_fulldata_g model, pushing the boundaries of digital image restoration and creative enhancement.
The places_512_fulldata_g model is an advanced image inpainting solution that excels at repairing and enhancing digital photos with high accuracy. Rooted in the robust Stable Diffusion framework, this model has been specifically tailored to meet the demands of image restoration tasks. Understanding its architecture, training, and optimal usage can help users leverage its full potential.
Detailed Overview of the places_512_fulldata_g Model Architecture
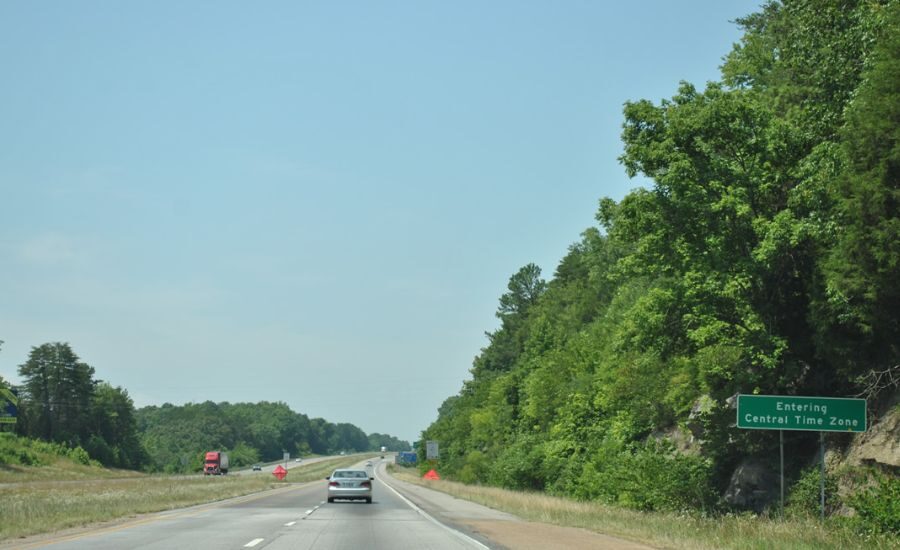
The places_512_fulldata_g model is an evolved version of the Stable Diffusion 1.5 architecture, adapted to specialize in inpainting tasks. It follows a comprehensive dual-stage training approach: an initial 595,000-step pre-training phase followed by an additional 440,000 steps focused on fine-tuning the model for inpainting at a resolution of 512×512 pixels. This method equips the model to effectively fill missing parts of an image while preserving visual and contextual integrity.
Core Architectural Design
Central to the places_512_fulldata_g model is a customized UNet architecture, which plays a vital role in its ability to fill in missing sections. Unlike traditional image generation models, this version features five extra input channels. Four channels handle the main image with missing content, while the fifth channel processes the mask that identifies the areas needing inpainting. This tailored input structure enables the model to manage masked regions efficiently.
Main Components:
Input Layer: Receives the original image and its mask.
Encoding Layers: Compress and process the image data.
UNet Core: Conducts the inpainting operations, filling in the missing parts.
Decoding Layers: Upscales and enhances the image for high-quality output.
Output Layer: Produces the final inpainted image, seamlessly blending restored areas with the original content.
Advantages Over Conventional Image Models
The places_512_fulldata_g model boasts several advantages for inpainting tasks when compared to general image generation models:
Improved Contextual Awareness: Trained on both complete and incomplete images, this model can better understand the context of the image, making it adept at maintaining the original composition during inpainting.
Smooth Edge Integration: It creates seamless transitions at the mask boundaries, reducing the visibility of where the inpainting was applied.
Enhanced Prompt Interpretation: The model’s specialized training allows it to accurately respond to prompts related to specific inpainting areas, ensuring more precise results.
Outpainting Functionality: While its primary focus is on inpainting, the model also handles outpainting—extending an image beyond its original borders with clarity and detail.
Preparing Images for Effective Inpainting
For the places_512_fulldata_g model to work best, images must be properly prepared. This includes several essential steps:
Image Preprocessing
Resizing: Standardizing photographs to a decision of 512×512 pixels ensures compatibility with the model’s education setup.
Normalization: Adjusting pixel depth values to a variety between zero and 1 improves the model’s performance and output consistency.
Contrast Enhancement: Techniques like histogram equalization may be applied to decorate the contrast in poorly lit photos, making it simpler for the version to recognize and inpaint info efficaciously.
Mask Creation and Refinement
Creating exquisite mask is important for successful inpainting. Users can use photograph modifying equipment to draw and modify mask. Using the mask blur slider, the precision of the mask’s edges can be customized, with better blur values growing smoother transitions between the inpainted vicinity and the authentic photo. For complicated sections, inclusive of specific textures or small capabilities, the “inpainting at Full Resolution” alternative is suggested for sharper, more accurate consequences.
Handling Various Image Resolutions
The places_512_fulldata_g model, trained at 512×512 pixels, also adapts well to higher resolutions, including up to 2K. While processing images at larger resolutions is possible, it can increase computation time. Users should consider this balance when working on projects that require detailed outputs without sacrificing processing efficiency.
Optimizing GPU Performance for Faster Results
Efficient use of GPU resources is crucial for speeding up inpainting tasks. Several strategies can enhance GPU performance:
Mixed-Precision Training: Utilizing both 32-bit and 16-bit floating-point formats can enhance computational speed and reduce memory usage without sacrificing accuracy. This allows for larger batch sizes, boosting GPU efficiency.
Data Transfer Optimization: To reduce latency, data transfer between the CPU and GPU should be streamlined. Techniques like CPU-pinned memory and caching frequently accessed data can aid in this.
NVIDIA DALI Library: This tool can be used to build optimized data processing pipelines, enabling offloading of specific tasks to the GPU and improving overall efficiency.
Balancing Speed and Output Quality
Achieving a balance between processing speed and image quality is essential. Users should fine-tune parameters such as layer height and processing time to meet specific needs. For instance, maintaining a high-resolution output might require longer processing, but it ensures higher-quality results. Lowering the layer height can help achieve finer details, though it may extend the time required for inpainting.
Facts:
- Model Name and File: The places_512_fulldata_g model is housed within the places_512_fulldata_g.pth file and is specifically built for advanced image inpainting tasks.
- Core Architecture: It is based on the Stable Diffusion 1.5 architecture with modifications, using a custom UNet design.
- Training Phases: The model underwent a two-phase training process—595,000 steps of general training followed by 440,000 steps focused on inpainting—at a resolution of 512×512 pixels.
- Five input channels are included, four of which are used for the picture data and one for the mask. This allows for detailed inpainting.
- Applications: Ideal for digital image restoration, photo editing, and creative design projects.
- Resolution Handling: While trained at 512×512 pixels, the model can work with higher resolutions up to 2K, balancing quality and processing time.
- Performance Optimization: Techniques include mixed-precision training, CPU-GPU data transfer improvements, and NVIDIA DALI library use.
- Post-Processing: Denoising methods like Gaussian blur can enhance image clarity, but techniques such as binarization and erosion may be less effective.
Summary
The places_512_fulldata_g model is an advanced tool for image inpainting, enabling users to reconstruct missing or damaged areas with high precision. Built on the Stable Diffusion 1.5 architecture and modified for inpainting, it processes images using a unique UNet architecture with added input channels for image and mask data. The model’s two-step training process enhances its ability to maintain context and create seamless, natural-looking results. It is versatile, able to handle images at resolutions up to 2K, though larger images may require more processing time. Key strategies for optimal use include image preprocessing, mask creation, and GPU optimization. Post-processing techniques can further refine outputs, enhancing detail and minimizing noise.
FAQs
Q1: What makes the places_512_fulldata_g model unique?
- The model is unique due to its specialized training on inpainting tasks and its adapted UNet architecture, which includes additional input channels for handling masks. This allows for higher accuracy in reconstructing missing parts while maintaining contextual relevance.
Q2: What is the recommended image size for optimal inpainting?
- The model is trained on 512×512 pixel images. While it can handle larger resolutions (up to 2K), processing time increases with the image size. Resizing images to 512×512 pixels ensures compatibility with the model’s training configuration.
Q3: How can I create effective masks for inpainting?
- Effective masks can be created using image editing tools. Adjusting the mask blur slider helps create smoother transitions between inpainted and original areas. For more detailed sections, such as small or intricate objects, using the “inpainting at Full Resolution” feature can improve accuracy.
Q4: How can I optimize GPU performance for faster inpainting?
- Strategies include using mixed-precision training (32-bit and 16-bit formats) to improve computational speed, optimizing data transfer between CPU and GPU with techniques like CPU-pinned memory and caching, and utilizing the NVIDIA DALI library to offload specific tasks to the GPU.
Q5: What post-processing methods can enhance inpainting results?
- Denoising methods such as Gaussian blur or median filters can be used to reduce noise and improve clarity. However, techniques like binarization and erosion are generally not recommended, as they may not enhance quality effectively. Combining multiple post-processing methods can lead to better results, but it’s crucial to test their impact on specific images.
Q6: Can the model handle large-scale image projects?
- Yes, the places_512_fulldata_g model can work with larger images, up to 2K resolution. However, processing larger images can result in longer processing times, so users should balance image resolution with processing efficiency.
Q7: What are the key advantages of using the places_512_fulldata_g model over general image generation models?
- The model excels in maintaining contextual integrity, seamlessly blending inpainted areas with the original image, and accurately responding to user prompts related to inpainting. It also has outpainting capabilities, extending images beyond their original borders with high detail.
For more Information About information visit Shortthink
Leave a Reply