Introduction To Places_512_Fulldata_G 目录
The places_512_fulldata_g listing has revolutionized the sphere of photograph inpainting through providing a powerful device for boosting and restoring virtual photos. This advanced model, on hand via the places_512_fulldata_g.Pth route, has end up integral for both experts and hobbyists. It gives a sturdy strategy to seamlessly fill in missing or damaged quantities of pix with outstanding precision. By know-how the context of an photograph and producing sensible content, this device has emerged as a transformative asset across industries which includes pictures, virtual recovery, and beyond.
This guide is designed to assist readers efficiently make use of the places_512_fulldata_g directory. It delves into the structure of the model, explaining how it procedures and interprets photo information. Readers will gain insights into making ready photographs for inpainting, making sure ideal consequences. Additionally, the object explores strategies to decorate performance and output excellent, allowing customers to completely leverage the capacity of this effective tool. By the stop, readers may have a complete know-how of how to maximize the skills of places_512_fulldata_g for their creative and professional tasks.
Understanding the places_512_fulldata_g Model for Image Inpainting
The places_512_fulldata_g model, along with its associated places_512_fulldata_g.pth path, is an advanced inpainting architecture designed to restore and enhance digital images with exceptional accuracy. Its innovative structure is built on the Stable Diffusion 1.5 framework, fine-tuned specifically for inpainting tasks, making it a critical tool for professionals and enthusiasts alike.
Core Model Architecture
The places_512_fulldata_g model’s foundation lies in its ability to handle masked regions within images, filling them with realistic and contextually appropriate content. Two stages of training are used to accomplish this:
Standard Training: The model undergoes approximately 595,000 steps to develop a general understanding of image data.
Inpainting-Specific Training: An additional 440,000 steps are devoted to training at a resolution of 512×512 pixels, focusing on filling in missing or masked areas seamlessly.
This dual-phase training equips the model with the ability to understand the nuances of both complete images and partially obscured sections, enabling it to reconstruct visually cohesive outputs.
Key Features of the Architecture
At the core of the places_512_fulldata_g model is a modified UNet architecture, optimized for inpainting tasks. Its unique enhancements include:
Additional Input Channels: Unlike traditional image generation models, this version features five extra input channels. Four channels encode the masked image, while the fifth encodes the mask itself, enabling a deeper understanding of both the image’s existing structure and the areas requiring restoration.
Layered Design:
Input Layer: Accepts both the original image and its mask.
Encoding Layers: Break down and analyze image data.
UNet Core: Processes the primary inpainting tasks.
Decoding Layers: Reconstruct and refine the image.
Output Layer: Produces the final, restored image.
Advantages Over Traditional Inpainting Models
The places_512_fulldata_g model excels in several areas:
Contextual Understanding: Its training on both complete and masked images enables it to preserve the context and aesthetics of the original image.
Seamless Edge Integration: The model reduces the visibility of masked edges, resulting in smoother transitions between restored and original areas.
Prompt Comprehension: It handles specific user inputs effectively, delivering precise and context-aware inpainting results.
Outpainting Capabilities: Beyond filling in gaps, the model can extend the boundaries of an image, maintaining consistency with its content.
Preparing Images for Optimal Results
Achieving high-quality outcomes with the places_512_fulldata_g model requires careful image preparation and masking.
Image Preprocessing
Resizing: Images should be resized to 512×512 pixels to align with the model’s training specifications.
Normalization: Adjust pixel depth values to a 0-1 variety to improve the model’s overall performance.
Contrast Enhancement: Techniques like histogram equalization can improve the visibility of information in poorly lit or low-evaluation pictures.
Effective Masking
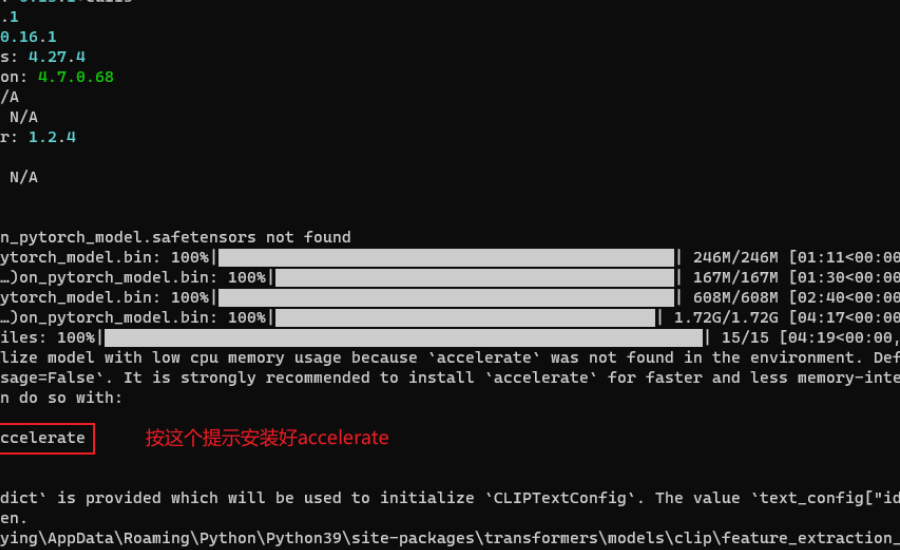
Creating specific masks is important for a hit inpainting. Utilize enhancing gear to define the masked place and adjust the masks blur to ensure smooth transitions. For complicated info, allowing “inpainting at full decision” lets in for zoomed-in processing, enhancing accuracy.
Handling High Resolutions
Although skilled on 512×512 snap shots, the model plays successfully with better resolutions, even as much as 2K. To stability best and processing time, users ought to test with resolution settings that in shape their specific needs.
Enhancing Performance and Quality
Optimizing GPU Usage
Leveraging GPU strength can extensively boost up the version’s performance:
Mixed-Precision Training: Utilize sixteen-bit floating-point computations to lessen reminiscence usage and growth velocity without compromising accuracy.
Optimized Data Transfer: Employ techniques like CPU-pinned reminiscence or NVIDIA DALI to streamline information transfer among the CPU and GPU.
Balancing Speed and Quality
Finding the right balance between speed and output quality is crucial. Adjust settings like batch size, resolution, and layer height to meet the desired performance and aesthetic goals.
Post-Processing Techniques
Post-processing can refine the model’s outputs. Common techniques include:
Blurring: Median and Gaussian blur methods help improve the realism of the restored areas.
Noise Reduction: Effective denoising methods can enhance the clarity of the final image.
Layer Merging: Combining outputs from multiple processing methods can sometimes yield better results, though this may require experimentation.
Unlocking the Full Potential of the places_512_fulldata_g Model
With proper preparation, careful adjustments, and strategic optimizations, users can harness the places_512_fulldata_g model to achieve outstanding results in image inpainting. Whether restoring old photographs or enhancing creative projects, this tool opens up endless possibilities for digital image enhancement.
Comprehensive Guide to the places_512_fulldata_g Model: Architecture, Optimization, and Use Cases
A potent tool for digital image inpainting is the places_512_fulldata_g model and its related places_512_fulldata_g.pth. Built on the highly versatile Stable Diffusion 1.5 architecture, this model has been fine-tuned to excel at reconstructing missing or corrupted parts of images while maintaining exceptional accuracy and contextual integrity.
Technical Foundation of the Model
The places_512_fulldata_g model employs a two-phase training methodology to achieve robust inpainting capabilities:
- Standard Training: The model underwent 595,000 steps of general training to build a foundational understanding of image generation.
- Inpainting-Specific Training: Subsequently, it completed 440,000 steps focusing on inpainting tasks, all at a resolution of 512×512 pixels.
This dual-stage process equips the model to seamlessly comprehend both complete images and the intricacies of filling missing or masked areas.
Core Architecture and Key Components
At the heart of the places_512_fulldata_g model lies an enhanced UNet architecture tailored for inpainting. Key features include:
- Extended Input Channels: The model incorporates five additional input channels—four for encoding the masked image and one for the mask itself. This enables the processing of both existing image content and masked regions.
- Core Layers:
- Input Layer: Processes the original image and mask.
- Encoding Layers: Compress and downsample image data.
- UNet Core: Handles inpainting computations with precision.
- Decoding Layers: Refine and upscale the inpainted image.
- Output Layer: Generates the final, restored image.
Preprocessing Images for Inpainting
To ensure optimal results, preparing images for the places_512_fulldata_g model requires specific preprocessing steps:
1. Image Resizing
All input images should be resized to a standard resolution of 512×512 pixels during both training and application. Uniform sizing allows the model to process data efficiently and consistently.
2. Normalization
Normalize pixel intensity values to a range between 0 and 1 to enhance the model’s performance. Additional techniques like histogram equalization can improve contrast and overall image quality.
3. Mask Creation
Masking is integral to inpainting. Tools such as image editors or integrated masking interfaces allow users to define specific regions. Adjust the mask edge precision using features like the mask blur slider, which ensures smooth transitions between the original and inpainted sections.
4. Handling Higher Resolutions
Though designed for 512×512 pixels, the model generalizes well for higher resolutions, including 2K images. Users should balance quality and processing time by experimenting with different resolution settings.
Comparison with Other Inpainting Models
The places_512_fulldata_g model outperforms many traditional generation models in key areas:
- Contextual Understanding: Its training on both complete and masked images enables superior contextual awareness.
- Edge Seamlessness: Produces smooth transitions with minimal artifacts along masked edges.
- Precision: Enhanced prompt comprehension ensures more accurate additions to the image.
- Outpainting Capabilities: Excels at extending images beyond their original boundaries.
Optimizing GPU Utilization for Performance
Efficient use of computational resources is crucial when working with the places_512_fulldata_g model:
- Mixed-Precision Training:
- Use mixed floating-point types (e.g., FP16 and FP32) to reduce memory requirements without compromising accuracy.
- This approach enables larger batch sizes and accelerates processing.
- Data Transfer Optimization:
- Employ CPU-pinned memory and tools like NVIDIA’s Data Loading Library (DALI) to speed up data transfers from CPU to GPU.
- Monitoring GPU Metrics:
- Track GPU utilization, memory consumption, and power usage to identify bottlenecks and improve efficiency.
Balancing Speed and Quality
Maintaining a balance between output quality and processing speed is essential:
- Layer Heights: For high-quality outputs, a layer height of 60-100 microns is optimal. Thinner layers (<60 microns) may offer better resolution but significantly increase processing time.
- Temperature Control: Adjust parameters such as nozzle temperatures between 205–210°C and print speeds at 50mm/s to optimize results in physical simulations or related tasks.
Enhancing Output with Post-Processing
Post-processing methods can refine the quality of inpainted images:
- Denoising: Techniques like Gaussian blur or median filters can smooth out inconsistencies.
- Combining Methods: Employing multiple post-processing steps often yields improved results, but users should evaluate combinations carefully to avoid diminishing returns.
Facts:
- Model Overview:
- The places_512_fulldata_g is an advanced image inpainting model, based on the Stable Diffusion 1.5 framework.
- A dual-phase training strategy is used to fine-tune it: 440,000 steps of inpainting-specific training at 512×512 resolution and 595,000 steps of conventional training.
- Key Features:
- Incorporates a modified UNet architecture with five additional input channels for processing masked images.
- Offers contextual understanding, ensuring visually coherent reconstructions and smooth integration between inpainted and original sections.
- Supports outpainting, enabling image extension beyond original boundaries.
- Image Preparation:
- Input images are resized to 512×512 pixels.
- Preprocessing steps include normalization and contrast enhancement (e.g., histogram equalization).
- Accurate masking is critical for achieving high-quality results.
- Resolution Handling:
- While optimized for 512×512 images, it can handle higher resolutions (up to 2K), balancing processing time and quality.
- Performance Optimization:
- Mixed-precision training (FP16 and FP32) improves GPU efficiency and reduces memory usage.
- Tools like NVIDIA Data Loading Library (DALI) streamline data transfer between CPU and GPU.
- Post-Processing:
- Methods like Gaussian blur, median filtering, and denoising enhance image quality.
- Combining multiple post-processing techniques can further improve results, though overuse might diminish returns.
- Applications:
- Useful for professionals in photography, digital restoration, and creative projects.
Summary
The places_512_fulldata_g model is an advanced inpainting tool designed to fill in missing or damaged parts of images with high accuracy. Built on the Stable Diffusion 1.5 framework, it features a dual-phase training process, enabling it to understand both complete and masked images. Key architectural advancements include a modified UNet structure with additional input channels for enhanced image context comprehension.
Preparation for optimal use involves resizing images to 512×512 pixels, applying normalization, and creating precise masks. The model can generalize to higher resolutions and offers features like outpainting, where images are extended beyond their original boundaries. Performance optimization is achieved through mixed-precision training and efficient GPU utilization techniques. Post-processing methods such as denoising and blurring further enhance output quality.
This model is widely applicable across industries, including digital restoration, creative projects, and professional photography, offering a balance between speed, quality, and efficiency.
FAQs
1. What is the places_512_fulldata_g model used for?
The model is used for image inpainting, restoring missing or damaged portions of images while maintaining contextual and visual integrity. It also supports outpainting, extending images beyond their original boundaries.
2. What are the model’s unique features?
- A modified UNet architecture with additional input channels for processing masked areas.
- Dual-phase training (standard and inpainting-specific) for detailed image reconstruction.
- Contextual understanding and seamless edge integration.
3. What resolution does the model support?
It is optimized for 512×512 pixels but can generalize effectively to higher resolutions, including 2K images.
4. How do I prepare images for inpainting?
- Resize images to 512×512 pixels.
- Normalize pixel intensity values to the 0-1 range.
- Use precise masks to define areas for inpainting and adjust mask blur for smooth transitions.
5. How can I optimize GPU usage?
- Use mixed-precision training (e.g., FP16 and FP32) for better performance and reduced memory use.
- Employ tools like NVIDIA DALI for efficient data transfers.
6. What post-processing techniques enhance results?
- Blurring methods (e.g., Gaussian and median filters) to smooth inconsistencies.
- Noise reduction techniques to improve clarity.
- Combining multiple post-processing steps to refine output, though caution is needed to avoid overprocessing.
7. Is this model suitable for beginners?
Yes, with proper guidance, the model can be used effectively by both professionals and hobbyists, thanks to its user-friendly architecture and detailed documentation.
For more Information About information visit Shortthink
Leave a Reply